Machine Learning is Silver Bullet for Cyber Security
Mark Leo 12/06/2024 10:30 114
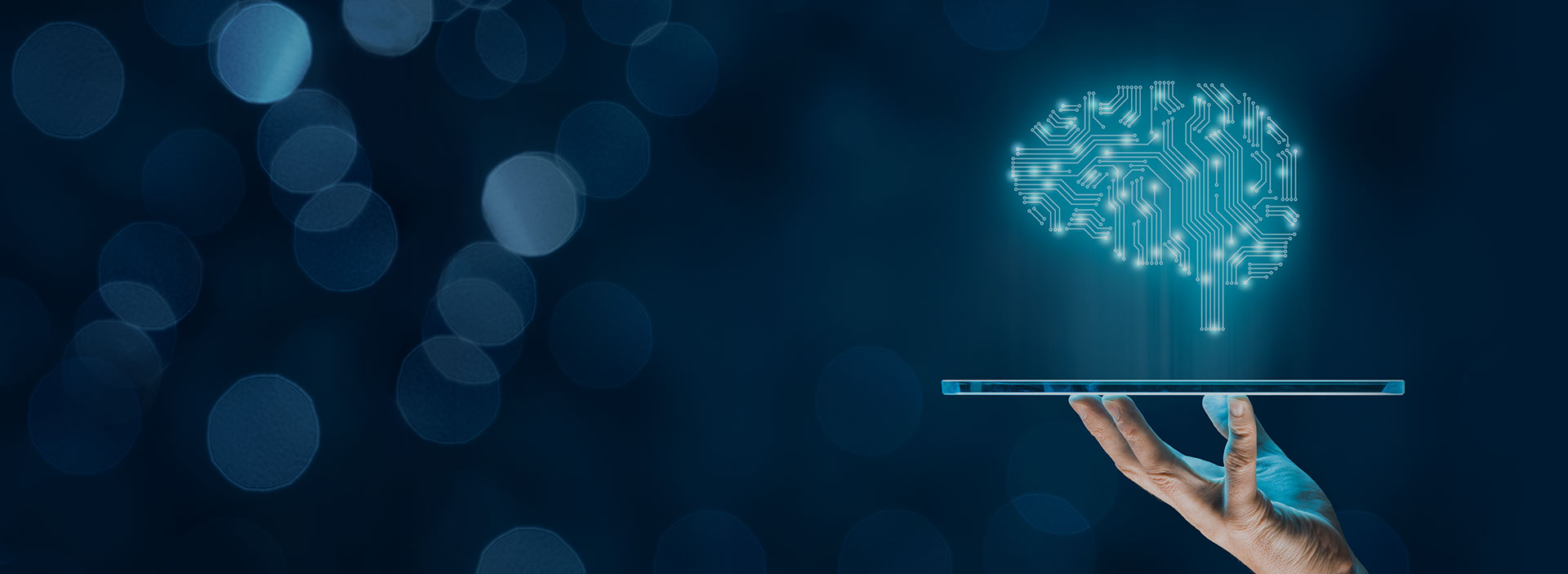
Seeing the exponential hike in global cyber threat spectrum, organizations are now striving for better data mining techniques in order to analyse security logs received from their IT infrastructures to ensure potent cyber threat detection and subsequent incident response. Machine Learning based analysis for security machine data is the next emerging trend in cyber security, aimed at minimizing the operational overheads of maintaining conventional static correlation rules in the security-monitoring devices. However, selecting the optimal algorithm with least number of false-positives still remains the impeding factor against the success of data science, especially in the case of any large-scale and global level Security Operations Centre (SOC) environment. This fact brings a dire need for an effective and efficient machine learning based cyber threat detection model. In this research, we are proposing optimal machine learning algorithms for detecting multiple types of threat actors by analytically and empirically comparing gathered results from various anomaly detection, classification and forecasting algorithms.
Machine Learning Organizational Strategy
Many organizations are now hitting physical limits with traditional approaches and technologies to collect, parse, normalize, search, analyze, visualize and explore the huge volume of security events collected by Security Information and Management Systems (SIEM). Traditional SIEM devices in any Security Operations Center (SOC) are designed to statically correlate security events and generate alerts for potential cyber threats. Most SIEM systems rely on these static threats correlation rules to report security incidents and kick-start the Incident Response (IR); but these correlation rules lack context and memory of normal behavioral baselines, and cause significant operational overheads to maintain, and thus falling short for providing real-time data mining capability that is of crucial importance to detect advanced and complex cyber-attacks.
A typical enterprise network with thousands of IT systems, generates billions of security events per day. Generally, only a subset of these security events are ingested by SIEMs to run through threat correlation rules, however organizations are now aiming to ingest as much security events as possible to meet regulatory requirements, and to leverage this security big data to enhance investigative visibility, conduct Threats Hunting (TH) and expedite the Incident Response (IR) process through live and historical analysis of security events. This trend has made the situation more worst and unmanageable by SOC Analysts in large-scale Security Operations Centers.
Therefore, organizations have now realized that the traditional monitoring complemented by an effective and versatile Machine Learning based Threat Hunting will be a necessary part of any Security Monitoring portfolio. Detection of complex cyber-attacks due to its noisy security log data will require utilization of classification, regression and forecasting algorithms to spot anomalies and detection new patterns to cater cyber-based as well as insider threats.
Nevertheless, Machine Learning based security analytics should be performed through an Optimal Workflow (as shown in figure-1) in order to ensure efficient preprocessing of data before applying a nicely trained machine learning predictor or classifier for subsequent analytics. Such a workflow can help in addressing all challenges by reducing the huge volume of security events to a few outlier events and providing security analysts with potential indicators of malicious activities to feed into cyber threats detection and hunting processes. Different algorithms work best for different security events types, and analysts should choose.